AI For Predictive Analytics
Predictive analytics is a vital aspect of AI, utilizing historical data patterns to derive outcomes for new scenarios. Generative AI enhances predictive analytics by imitating data distributions and creating robust models for accurate predictions.
In the realm of AI for predictive analytics, tools like Salesforce Einstein and Clari stand out for their effective forecasting capabilities, leveraging machine learning to analyze sales data and provide real-time insights. These AI-driven tools play a crucial role in improving decision-making processes and driving business success through data-driven forecasting.
AI For Predictive Analytics

The Rise of AI In Predictive Analytics
AI for predictive analytics has seen a remarkable rise, leveraging historical data patterns, hidden insights, and data distribution to derive outcomes for newly occurring scenarios. Generative AI complements predictive analytics by imitating data distribution and creating robust models, enhancing the prediction process.
AI Revolutionizing Predictive Analytics
AI has revolutionized the field of predictive analytics, offering advanced tools and technologies that enable businesses to make accurate forecasts and data-driven decisions.
Benefits Of Using AI In Predictive Analytics
Using AI for predictive analytics comes with a myriad of benefits, including enhanced accuracy, faster insights, improved decision-making, and the ability to uncover hidden patterns in data.
Key Techniques In AI For Predictive Analytics
Key Techniques in AI for Predictive Analytics encompass a range of advanced methods that enable businesses to forecast future events and trends with greater accuracy. Leveraging the power of artificial intelligence, predictive analytics has become an indispensable tool for decision-making and strategic planning. Let’s delve into the key techniques that underpin AI for predictive analytics.
Machine Learning Algorithms- AI For Predictive Analytics
Machine learning algorithms are the cornerstone of predictive analytics, enabling systems to learn from data and make predictions or decisions. These algorithms include supervised learning, unsupervised learning, and reinforcement learning, each serving different purposes in predictive analytics.
Deep Learning Models
Deep learning models, a subset of machine learning, involve neural networks with multiple layers that can automatically discover representations from data. They are particularly effective for handling unstructured data such as images, audio, and text, making them a powerful tool for predictive analytics.
Natural Language Processing
Natural Language Processing (NLP) is a key technique in AI for predictive analytics, enabling systems to understand, interpret, and generate human language. NLP plays a crucial role in sentiment analysis, text classification, and language translation, providing valuable insights for predictive modeling.
Challenges and Limitations
Predictive analytics powered by AI faces challenges and limitations, including the need for large, high-quality data sets, potential biases in the data, and the complexity of interpreting results. Implementing AI for predictive analytics also requires skilled professionals and robust infrastructure to handle the computational demands.
Artificial Intelligence (AI) has revolutionized predictive analytics, enabling organizations to make data-driven decisions and gain valuable insights. However, despite its numerous benefits, AI for predictive analytics also presents several challenges and limitations that need to be addressed for optimal utilization.
Data Quality And Quantity
One of the primary challenges in AI for predictive analytics is ensuring the quality and quantity of data. Inadequate or poor-quality data can significantly impact the accuracy and reliability of predictive models. Additionally, the sheer volume of data generated can pose a challenge in terms of storage, processing, and analysis. It is essential to have robust data collection and cleansing processes to ensure that the data used for predictive analytics is of high quality and sufficient quantity to yield meaningful results.
Interpretability And Explainability
Another significant limitation of AI for predictive analytics is the lack of interpretability and explainability in the models. Complex AI algorithms may produce accurate predictions, but often lack transparency. This can be a concern, particularly in critical domains such as healthcare, where it is essential to understand the rationale behind AI-driven predictions. Ensuring that AI models are interpretable and explainable is crucial for gaining trust and acceptance from stakeholders and end-users.
Ethical Concerns
AI for predictive analytics also raises ethical concerns, especially regarding privacy, bias, and accountability. The use of sensitive personal data in predictive analytics, particularly in healthcare, requires stringent ethical considerations. Moreover, the potential for biases to be perpetuated or amplified by AI algorithms poses a significant ethical challenge. Addressing these ethical concerns is imperative to ensure that AI predictive analytics is used responsibly and ethically. In conclusion, while AI for predictive analytics offers remarkable potential, it is essential to acknowledge and address the challenges and limitations it presents. By overcoming these hurdles, organizations can harness the power of AI to drive impactful and ethical predictive analytics solutions.

Real-world Applications
Discover how AI for Predictive Analytics revolutionizes decision-making by analyzing historical data patterns and deriving insights for future scenarios. Generative AI enhances predictive models, creating robust outcomes and supporting efficient prediction processes. Experience the power of AI-driven analytics in optimizing business strategies and forecasting trends.
Real-World Applications AI for Predictive Analytics has revolutionized numerous industries, offering real-world applications that have transformed the way organizations make decisions. From healthcare to finance and e-commerce, the integration of AI for predictive analytics has led to significant advancements in data-driven decision-making processes. Healthcare In the healthcare sector, AI for predictive analytics has played a pivotal role in improving patient outcomes and optimizing healthcare operations. By analyzing vast amounts of patient data, AI algorithms can predict potential health risks, identify early signs of diseases, and personalize treatment plans. This has led to more efficient resource allocation and improved patient care. Finance In the finance industry, AI-powered predictive analytics has been utilized for fraud detection, risk assessment, and investment forecasting. By leveraging AI algorithms, financial institutions can identify patterns of fraudulent activities, assess credit risks, and make accurate predictions about market trends. This has resulted in enhanced security measures and more informed financial decision-making processes.
Best Practices For Implementing AI In Predictive Analytics
Implementing AI in predictive analytics involves leveraging generative AI models to analyze historical data patterns and hidden insights. By combining generative AI with predictive analytics, businesses can enhance their forecasting accuracy and generate potential scenarios for better decision-making. Embracing AI-driven predictive analytics can unlock new opportunities for businesses seeking to optimize their data analysis processes.
dictive analytics? There are several best practices to keep in mind when implementing AI in predictive analytics techniques to get the most out of your data. These practices include data preprocessing, model selection and evaluation, and continuous learning.
Data Preprocessing
Data preprocessing is the first step in developing a successful predictive AI model. It involves cleaning, transforming, and organizing the data to ensure that it is relevant and accurate. This can include removing any duplicates or inconsistencies, handling missing data, and scaling features to ensure that they are on the same level. By doing so, you can ensure that the AI model is working with the best possible data and minimize the risk of errors or inaccuracies in the predictions.
Model Selection And Evaluation
Model selection and evaluation are crucial steps in developing a successful predictive AI model. This involves selecting the right algorithm for the task at hand and evaluating its performance to ensure that it is accurate and reliable. It is important to choose a model that is appropriate for the data and the problem being solved. Additionally, it is essential to evaluate the model’s performance using appropriate metrics and testing techniques to ensure that it is performing as expected.
Continuous Learning
Continuous learning is an important aspect of predictive AI models as it allows them to adapt to changing circumstances and improve their accuracy over time. This involves regularly updating the model with new data and adjusting its parameters to ensure that it is performing at its best. By doing so, you can ensure that the model is always up-to-date and accurate, and that it continues to provide valuable insights and predictions. In conclusion, implementing AI in predictive analytics techniques can be a powerful tool for businesses looking to gain insights and make data-driven decisions. By following best practices such as data preprocessing, model selection and evaluation, and continuous learning, you can ensure that your predictive AI models are accurate, reliable, and provide valuable insights that can drive business success.
Future Trends In AI For Predictive Analytics
Discover the evolving landscape of AI for predictive analytics, shaping the future of data-driven insights. Generative AI models enhance predictive capabilities by mimicking data distributions, offering robust solutions for accurate forecasting and scenario planning. Explore how AI drives innovation in predictive analytics for smarter decision-making.
AI has become increasingly important in predictive analytics, and its importance is only expected to grow in the future. As we look ahead, we can see a number of trends emerging in the field of AI for predictive analytics. In this article, we’ll take a closer look at some of these trends, including automated machine learning (AutoML) and AI-powered decision support systems.
Automated Machine Learning (autumn)
One of the most significant trends in AI for predictive analytics is the rise of automated machine learning (AutoML). This technology is designed to make it easier for businesses to build predictive models without requiring extensive data science expertise. With AutoML, businesses can automate much of the process of building and tuning predictive models, allowing them to get results faster and with less effort. There are a number of different AutoML tools available today, each with its own strengths and weaknesses. Some are designed for specific industries, while others are more general-purpose. Regardless of the specific tool, however, the goal is the same: to make it easier for businesses to leverage AI for predictive analytics.
Ai-powered Decision Support Systems
Another important trend in AI for predictive analytics is the development of AI-powered decision support systems. These systems are designed to help businesses make better decisions by providing them with real-time insights and recommendations based on data. They can be used in a wide range of contexts, from healthcare to finance to marketing. One of the key advantages of AI-powered decision support systems is their ability to process large amounts of data quickly and accurately. They can analyze data from a variety of sources, including social media, customer feedback, and financial data, and provide businesses with actionable insights in real-time. In addition to these two trends, there are a number of other developments in AI for predictive analytics that are worth watching.
Ethical Considerations In AI-driven Predictive Analytics
Bias And Fairness
One of the significant ethical concerns in AI-driven predictive analytics is the potential for bias and fairness. Bias can arise from skewed datasets or inherent prejudices in the algorithms themselves. It’s essential to ensure that the predictive models are designed and trained in a way that minimizes bias and promotes fairness in decision-making processes.
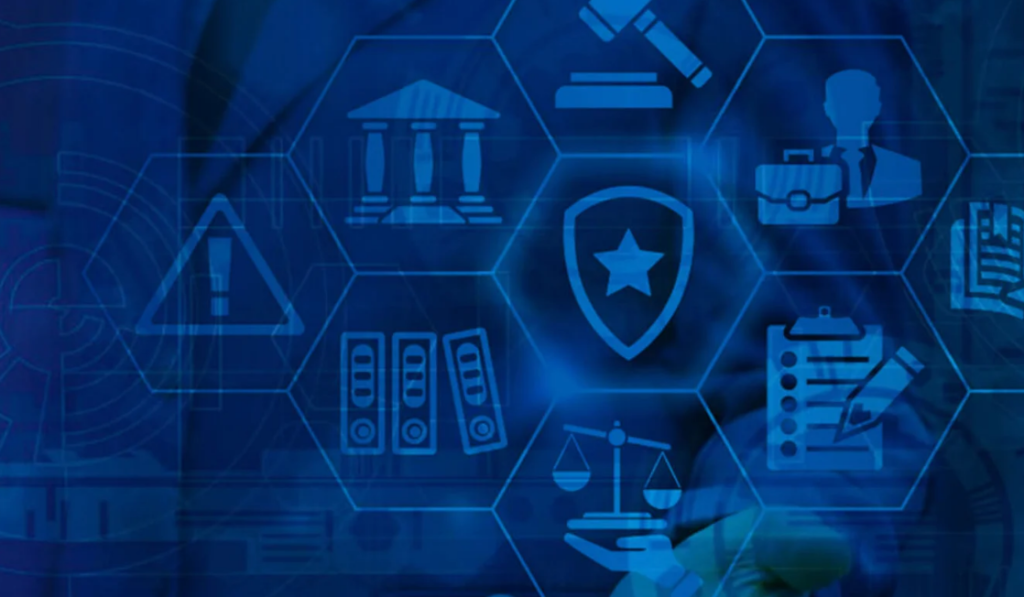
Privacy and Data Security
Another critical ethical consideration in AI-driven predictive analytics is privacy and data security. As predictive analytics heavily rely on vast amounts of data, it’s crucial to safeguard the privacy of individuals and ensure the security of sensitive information. Implementing robust data protection measures and adhering to privacy regulations are imperative to maintain trust and ethical standards.
Conclusion
In the realm of predictive analytics, AI offers unparalleled insights and forecasting capabilities. By leveraging generative AI models, businesses can enhance their predictive analytics strategies for more accurate predictions and improved decision-making. Embracing AI for predictive analytics opens up a world of possibilities for organizations seeking to stay ahead in a data-driven landscape.